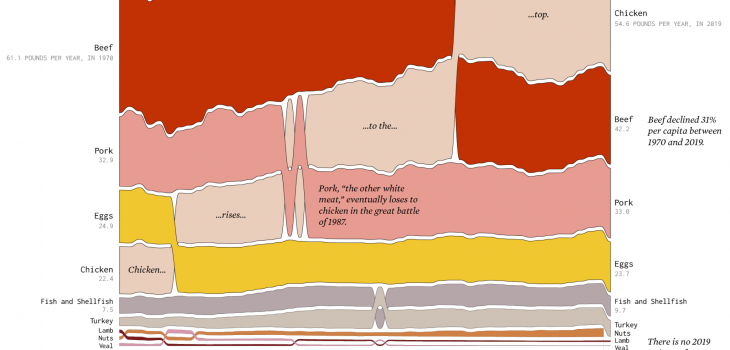
Why 3rd party research should not be a primary…
One mistake I find product managers often make is overuse or over weighting of third party research findings. While 3rd party research has its utility, it a) rarely asks the specific questions about what people need and b) looks backwards when you’re trying to find proxies for the future. Let’s look at an example.
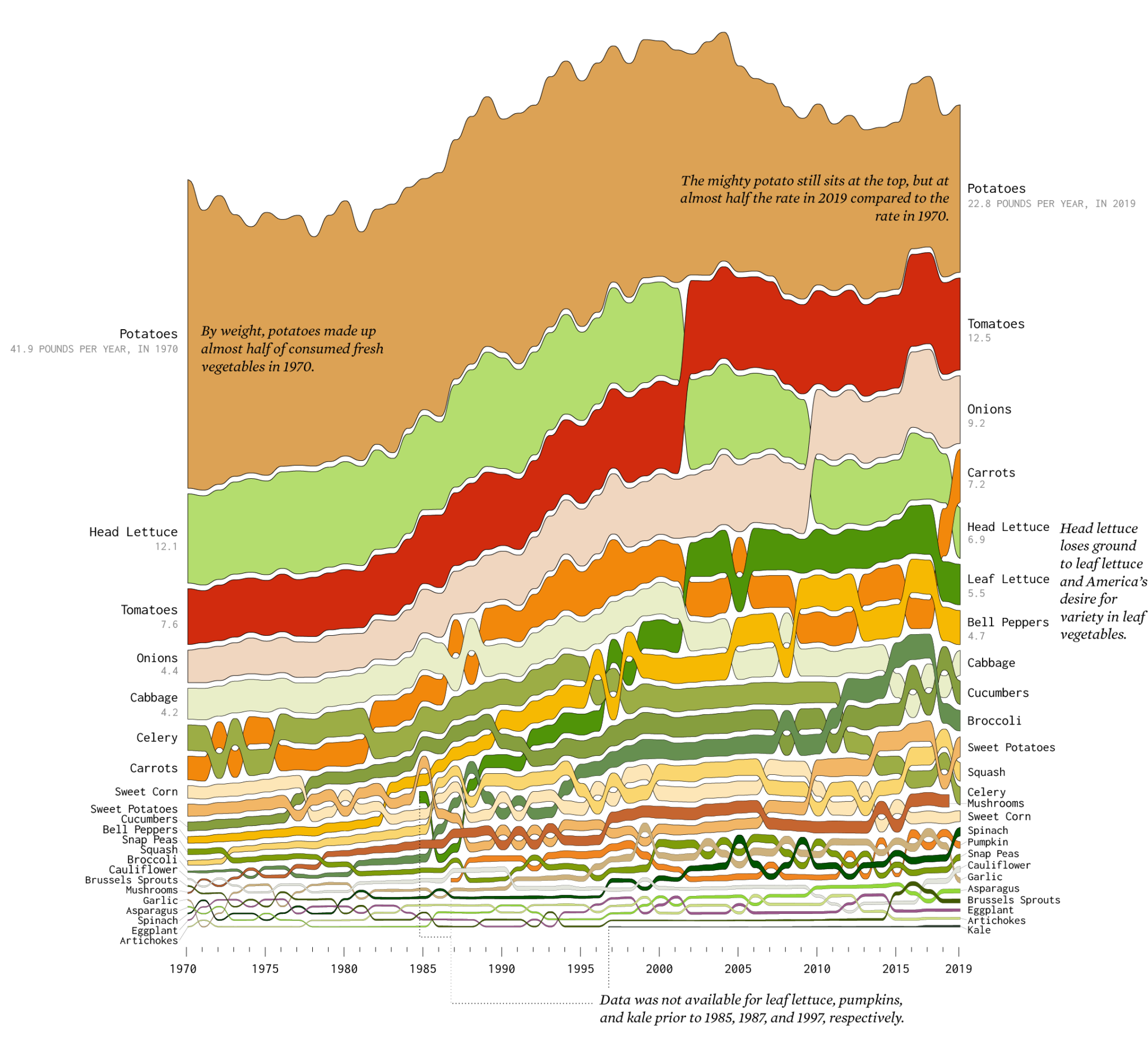
This recent infographic shows changes in food consumption behavior since 1970. There are several interesting insights including how chicken is now the most dominant protein (was beef) and leaf lettuce was non-existent as a concept in 1970. Diving deeper into the vegetable chart, one can see several other interesting changes. If you were a food products company (anywhere in the value chain) thinking about new products or even where capex spend might be applied, this chart might be a tempting input (let’s assume we trust the data source and veracity of the research).
However, while trend lines can be drawn (esp with the underlying data), this chart does not begin to answer “why” these changes have occurred. We could sit around a table and speculate about them: leaf lettuce was not transportable to market in 1970; recent health trends have people eating more salad; potatoes, while still popular, have historically been consumed in fried fashion which is not part of a modern, health conscious diet…and many more. Some of these might be spot on, some wild guesses. However, until we talk to customers about their eating trends (esp those who are older and have a longer history), we cannot accurately create the narrative this data shows, let alone create testable hypotheses as to how predictive these trends our for our new food product.
Data can point to problems or opportunities, qualitative research is how we start the search for solutions (predicting the future) through iterative experimentation.